Table of Contents
Machine learning (ML), a subset of artificial intelligence (AI), is revolutionizing healthcare by enhancing diagnostics, personalizing treatment, and streamlining operations. By leveraging algorithms that can learn from and make predictions based on data, ML is transforming various aspects of the healthcare industry, leading to improved patient outcomes, increased efficiency, and reduced costs. This article explores how machine learning is reshaping healthcare and the impact it is having on the field.
Enhancing Diagnostics
One of the most significant contributions of machine learning to healthcare is its role in improving diagnostic accuracy. Traditional diagnostic methods often rely on the expertise and experience of healthcare professionals, which can be limited by human error and subjectivity. Machine learning algorithms, however, can analyze vast amounts of data quickly and with high precision, leading to more accurate and consistent diagnoses.
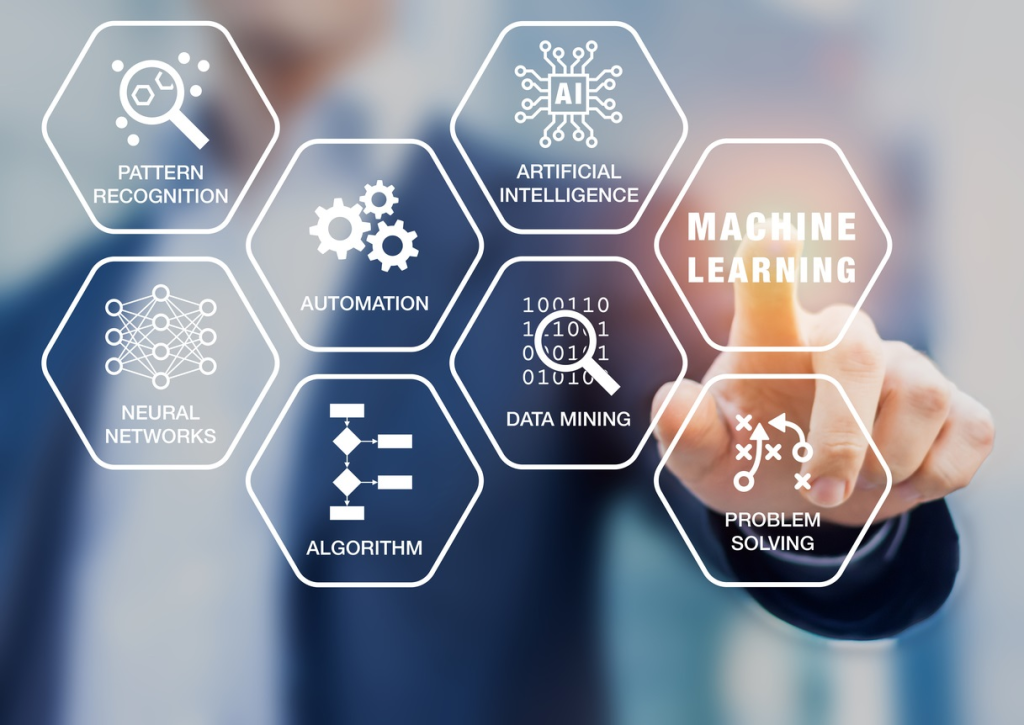
- Medical Imaging: ML algorithms have shown remarkable success in interpreting medical images, such as X-rays, MRIs, and CT scans. For instance, deep learning models can detect abnormalities such as tumors, fractures, or lesions with accuracy comparable to or even exceeding that of experienced radiologists. These models are trained on large datasets of annotated images, enabling them to identify patterns and anomalies that may be missed by the human eye. This not only enhances diagnostic accuracy but also speeds up the interpretation process, allowing for quicker patient management.
- Pathology: In pathology, ML is used to analyze tissue samples and detect diseases such as cancer. Algorithms can examine histopathological slides, identifying cancerous cells and classifying them into different types and stages. This helps pathologists make more precise diagnoses and develop tailored treatment plans.
Personalizing Treatment
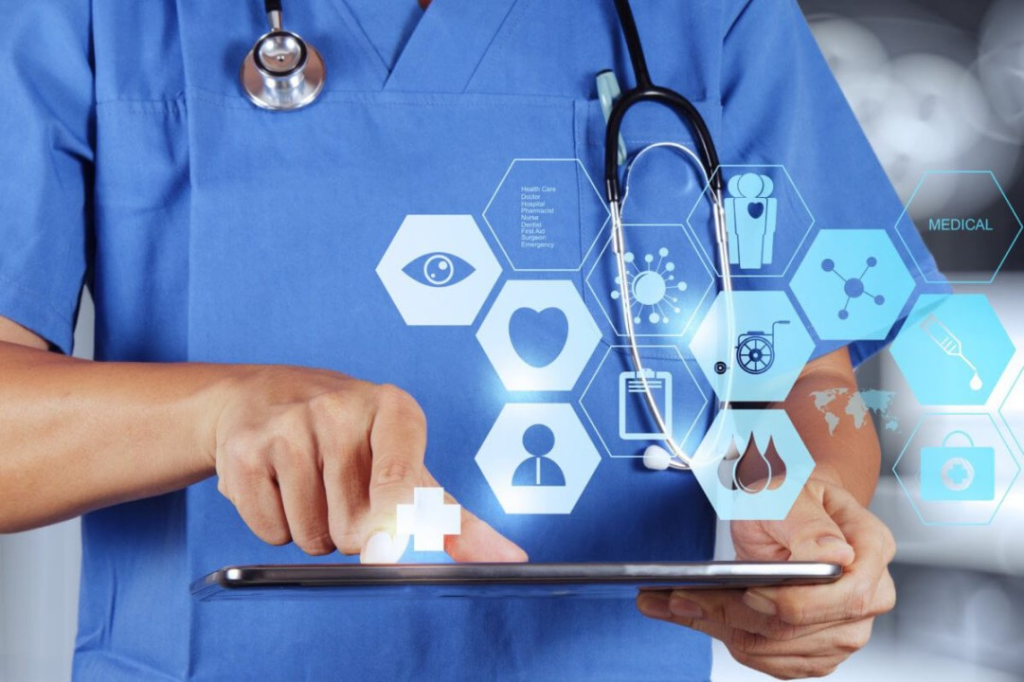
Machine learning is also transforming healthcare by enabling personalized treatment approaches. Rather than applying a one-size-fits-all model, ML algorithms can analyze patient data to tailor treatments to individual needs, improving effectiveness and reducing adverse effects.
- Precision Medicine: ML facilitates precision medicine by analyzing genetic, environmental, and lifestyle data to predict individual responses to treatments. For example, ML algorithms can analyze genomic data to identify specific genetic mutations that influence how patients respond to certain drugs. This information helps clinicians select the most effective treatments and avoid those that may be less effective or harmful.
- Drug Discovery and Development: ML accelerates drug discovery by analyzing vast datasets of chemical compounds, biological interactions, and clinical trial results. Algorithms can identify potential drug candidates, predict their effectiveness, and suggest modifications to improve their efficacy. This speeds up the development process and reduces the costs associated with bringing new drugs to market.
Streamlining Operations
Beyond diagnostics and treatment, machine learning is streamlining healthcare operations, leading to increased efficiency and cost savings.
- Predictive Analytics: ML algorithms can predict patient outcomes and resource needs by analyzing historical data. For instance, predictive models can forecast hospital readmissions, allowing healthcare providers to implement preventive measures and allocate resources more effectively. These models can also predict patient demand for services, helping hospitals manage staff levels and reduce wait times.
- Administrative Tasks: ML is automating administrative tasks, such as medical coding and billing. Algorithms can process electronic health records (EHRs) and extract relevant information for billing and coding purposes, reducing the administrative burden on healthcare providers and minimizing errors. This automation streamlines operations and allows healthcare professionals to focus more on patient care.
- Clinical Decision Support: ML-powered decision support systems assist clinicians in making informed decisions by providing evidence-based recommendations. These systems analyze patient data and medical literature to offer insights and suggest treatment options, helping clinicians make more informed and timely decisions.
Challenges and Considerations
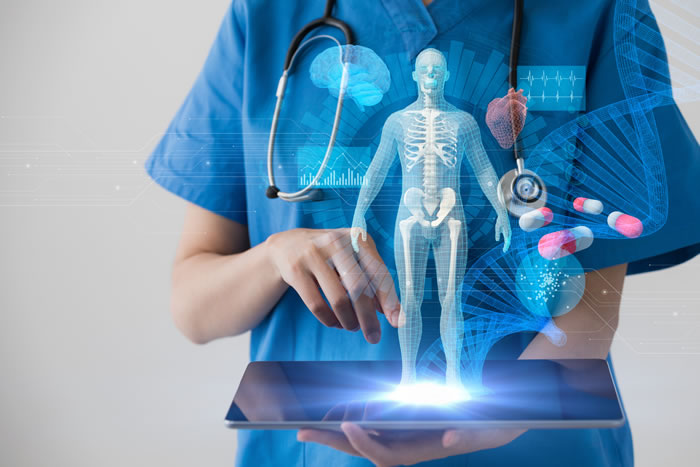
Despite its transformative potential, machine learning in healthcare also presents several challenges and considerations:
- Data Privacy and Security: The use of ML requires access to vast amounts of sensitive patient data. Ensuring the privacy and security of this data is paramount to maintaining patient trust and complying with regulations such as HIPAA (Health Insurance Portability and Accountability Act). Healthcare organizations must implement robust data protection measures and secure data-sharing protocols.
- Bias and Fairness: ML algorithms are only as good as the data they are trained on. If the training data is biased or unrepresentative, the algorithms may produce biased results, leading to disparities in healthcare outcomes. Ensuring that ML models are trained on diverse and representative datasets is crucial for achieving fairness and equity in healthcare.
- Integration and Adoption: Integrating ML technologies into existing healthcare systems can be challenging. Healthcare organizations must address technical, logistical, and financial barriers to successfully implement and adopt ML solutions. Training healthcare professionals to effectively use these technologies is also essential for maximizing their benefits.
Conclusion
Machine learning is profoundly transforming healthcare by enhancing diagnostic accuracy, personalizing treatment, and streamlining operations. Its applications are improving patient outcomes, accelerating drug discovery, and increasing efficiency within healthcare systems. However, addressing challenges related to data privacy, bias, and integration is crucial for realizing the full potential of ML in healthcare.
As machine learning technology continues to evolve, its role in healthcare is likely to expand, offering new opportunities for innovation and improvement. By leveraging ML effectively and responsibly, the healthcare industry can drive significant advancements in patient care, research, and operational efficiency, paving the way for a more effective and personalized healthcare system.